A latest examine from the US has discovered that the real-world efficiency of in style Retrieval Augmented Era (RAG) analysis techniques corresponding to Perplexity and Bing Copilot falls far wanting each the advertising hype and in style adoption that has garnered headlines over the past 12 months.
The venture, which concerned in depth survey participation that includes 21 skilled voices, discovered a minimum of 16 areas through which the studied RAG techniques (You Chat, Bing Copilot and Perplexity) produced trigger for concern:
1: A scarcity of goal element within the generated solutions, with generic summaries and scant contextual depth or nuance.
2. Reinforcement of perceived person bias, the place a RAG engine continuously fails to current a variety of viewpoints, however as an alternative infers and reinforces person bias, based mostly on the way in which that the person phrases a query.
3. Overly assured language, significantly in subjective responses that can’t be empirically established, which might lead customers to belief the reply greater than it deserves.
4: Simplistic language and a scarcity of vital pondering and creativity, the place responses successfully patronize the person with ‘dumbed-down’ and ‘agreeable’ data, as an alternative of thought-through cogitation and evaluation.
5: Misattributing and mis-citing sources, the place the reply engine makes use of cited sources that don’t assist its response/s, fostering the phantasm of credibility.
6: Cherry-picking data from inferred context, the place the RAG agent seems to be searching for solutions that assist its generated rivalry and its estimation of what the person desires to listen to, as an alternative of basing its solutions on goal evaluation of dependable sources (presumably indicating a battle between the system’s ‘baked’ LLM knowledge and the information that it obtains on-the-fly from the web in response to a question).
7: Omitting citations that assist statements, the place supply materials for responses is absent.
8: Offering no logical schema for its responses, the place customers can not query why the system prioritized sure sources over different sources.
9: Restricted variety of sources, the place most RAG techniques usually present round three supporting sources for an announcement, even the place a larger range of sources can be relevant.
10: Orphaned sources, the place knowledge from all or among the system’s supporting citations will not be truly included within the reply.
11: Use of unreliable sources, the place the system seems to have most popular a supply that’s in style (i.e., in search engine marketing phrases) moderately than factually appropriate.
12: Redundant sources, the place the system presents a number of citations through which the supply papers are basically the identical in content material.
13: Unfiltered sources, the place the system affords the person no solution to consider or filter the supplied citations, forcing customers to take the choice standards on belief.
14: Lack of interactivity or explorability, whereby a number of of the user-study members have been pissed off that RAG techniques didn’t ask clarifying questions, however assumed user-intent from the primary question.
15: The necessity for exterior verification, the place customers really feel compelled to carry out unbiased verification of the equipped response/s, largely eradicating the supposed comfort of RAG as a ‘substitute for search’.
16: Use of educational quotation strategies, corresponding to [1] or [34]; that is customary observe in scholarly circles, however might be unintuitive for a lot of customers.
For the work, the researchers assembled 21 consultants in synthetic intelligence, healthcare and drugs, utilized sciences and schooling and social sciences, all both post-doctoral researchers or PhD candidates. The members interacted with the examined RAG techniques while talking their thought processes out loud, to make clear (for the researchers) their very own rational schema.
The paper extensively quotes the members’ misgivings and issues in regards to the efficiency of the three techniques studied.
The methodology of the user-study was then systematized into an automatic examine of the RAG techniques, utilizing browser management suites:
‘A big-scale automated analysis of techniques like You.com, Perplexity.ai, and BingChat confirmed that none met acceptable efficiency throughout most metrics, together with vital facets associated to dealing with hallucinations, unsupported statements, and quotation accuracy.’
The authors argue at size (and assiduously, within the complete 27-page paper) that each new and skilled customers ought to train warning when utilizing the category of RAG techniques studied. They additional suggest a brand new system of metrics, based mostly on the shortcomings discovered within the examine, that would kind the muse of larger technical oversight sooner or later.
Nevertheless, the rising public utilization of RAG techniques prompts the authors additionally to advocate for apposite laws and a larger stage of enforceable governmental coverage in regard to agent-aided AI search interfaces.
The examine comes from 5 researchers throughout Pennsylvania State College and Salesforce, and is titled Search Engines in an AI Period: The False Promise of Factual and Verifiable Supply-Cited Responses. The work covers RAG techniques as much as the cutting-edge in August of 2024
The RAG Commerce-Off
The authors preface their work by reiterating 4 recognized shortcomings of Giant Language Fashions (LLMs) the place they’re used inside Reply Engines.
Firstly, they’re susceptible to hallucinate data, and lack the aptitude to detect factual inconsistencies. Secondly, they’ve problem assessing the accuracy of a quotation within the context of a generated reply. Thirdly, they have a tendency to favor knowledge from their very own pre-trained weights, and will resist knowledge from externally retrieved documentation, although such knowledge could also be more moderen or extra correct.
Lastly, RAG techniques have a tendency in the direction of people-pleasing, sycophantic habits, typically on the expense of accuracy of knowledge of their responses.
All these tendencies have been confirmed in each facets of the examine, amongst many novel observations in regards to the pitfalls of RAG.
The paper views OpenAI’s SearchGPT RAG product (launched to subscribers final week, after the brand new paper was submitted), as more likely to to encourage the user-adoption of RAG-based search techniques, despite the foundational shortcomings that the survey outcomes trace at*:
‘The discharge of OpenAI’s ‘SearchGPT,’ marketed as a ‘Google search killer’, additional exacerbates [concerns]. As reliance on these instruments grows, so does the urgency to know their influence. Lindemann introduces the idea of Sealed Information, which critiques how these techniques restrict entry to various solutions by condensing search queries into singular, authoritative responses, successfully decontextualizing data and narrowing person views.
‘This “sealing” of data perpetuates choice biases and restricts marginalized viewpoints.’
The Examine
The authors first examined their examine process on three out of 24 chosen members, all invited by means corresponding to LinkedIn or e mail.
The primary stage, for the remaining 21, concerned Experience Data Retrieval, the place members averaged round six search enquiries over a 40-minute session. This part targeting the gleaning and verification of fact-based questions and solutions, with potential empirical options.
The second section involved Debate Data Retrieval, which dealt as an alternative with subjective issues, together with ecology, vegetarianism and politics.
Generated examine solutions from Perplexity (left) and You Chat (proper). Supply: https://arxiv.org/pdf/2410.22349
Since the entire techniques allowed no less than some stage of interactivity with the citations offered as assist for the generated solutions, the examine topics have been inspired to work together with the interface as a lot as attainable.
In each instances, the members have been requested to formulate their enquiries each via a RAG system and a traditional search engine (on this case, Google).
The three Reply Engines – You Chat, Bing Copilot, and Perplexity – have been chosen as a result of they’re publicly accessible.
Nearly all of the members have been already customers of RAG techniques, at various frequencies.
As a consequence of house constraints, we can not break down every of the exhaustively-documented sixteen key shortcomings discovered within the examine, however right here current a choice of among the most attention-grabbing and enlightening examples.
Lack of Goal Element
The paper notes that customers discovered the techniques’ responses continuously lacked goal element, throughout each the factual and subjective responses. One commented:
‘It was simply attempting to reply with out truly giving me a strong reply or a extra thought-out reply, which I’m able to get with a number of Google searches.’
One other noticed:
‘It’s too brief and simply summarizes every part lots. [The model] wants to present me extra knowledge for the declare, nevertheless it’s very summarized.’
Lack of Holistic Viewpoint
The authors categorical concern about this lack of nuance and specificity, and state that the Reply Engines continuously didn’t current a number of views on any argument, tending to facet with a perceived bias inferred from the person’s personal phrasing of the query.
One participant stated:
‘I need to discover out extra in regards to the flip facet of the argument… that is all with a pinch of salt as a result of we don’t know the opposite facet and the proof and info.’
One other commented:
‘It isn’t supplying you with each side of the argument; it’s not arguing with you. As a substitute, [the model] is simply telling you, ’you’re proper… and listed below are the explanation why.’
Assured Language
The authors observe that every one three examined techniques exhibited using over-confident language, even for responses that cowl subjective issues. They contend that this tone will are inclined to encourage unjustified confidence within the response.
A participant famous:
‘It writes so confidently, I really feel satisfied with out even trying on the supply. However once you have a look at the supply, it’s unhealthy and that makes me query it once more.’
One other commented:
‘If somebody doesn’t precisely know the suitable reply, they may belief this even when it’s unsuitable.’
Incorrect Citations
One other frequent drawback was misattribution of sources cited as authority for the RAG techniques’ responses, with one of many examine topics asserting:
‘[This] assertion doesn’t appear to be within the supply. I imply the assertion is true; it’s legitimate… however I don’t know the place it’s even getting this data from.’
The brand new paper’s authors remark †:
‘Contributors felt that the techniques have been utilizing citations to legitimize their reply, creating an phantasm of credibility. This facade was solely revealed to some customers who proceeded to scrutinize the sources.’
Cherrypicking Data to Go well with the Question
Returning to the notion of people-pleasing, sycophantic habits in RAG responses, the examine discovered that many solutions highlighted a specific point-of-view as an alternative of comprehensively summarizing the subject, as one participant noticed:
‘I really feel [the system] is manipulative. It takes just some data and it feels I’m manipulated to solely see one facet of issues.’
One other opined:
‘[The source] truly has each execs and cons, and it’s chosen to choose simply the kind of required arguments from this hyperlink with out the entire image.’
For additional in-depth examples (and a number of vital quotes from the survey members), we refer the reader to the supply paper.
Automated RAG
Within the second section of the broader examine, the researchers used browser-based scripting to systematically solicit enquiries from the three studied RAG engines. They then used an LLM system (GPT-4o) to research the techniques’ responses.
The statements have been analyzed for question relevance and Professional vs. Con Statements (i.e., whether or not the response is for, in opposition to, or impartial, in regard to the implicit bias of the question.
An Reply Confidence Rating was additionally evaluated on this automated section, based mostly on the Likert scale psychometric testing technique. Right here the LLM choose was augmented by two human annotators.
A 3rd operation concerned using web-scraping to acquire the full-text content material of cited web-pages, via the Jina.ai Reader software. Nevertheless, as famous elsewhere within the paper, most web-scraping instruments are not any extra in a position to entry paywalled websites than most individuals are (although the authors observe that Perplexity.ai has been recognized to bypass this barrier).
Further issues have been whether or not or not the solutions cited a supply (computed as a ‘quotation matrix’), in addition to a ‘factual assist matrix’ – a metric verified with the assistance of 4 human annotators.
Thus 8 overarching metrics have been obtained: one-sided reply; overconfident reply; related assertion; uncited sources; unsupported statements; supply necessity; quotation accuracy; and quotation thoroughness.
The fabric in opposition to which these metrics have been examined consisted of 303 curated questions from the user-study section, leading to 909 solutions throughout the three examined techniques.
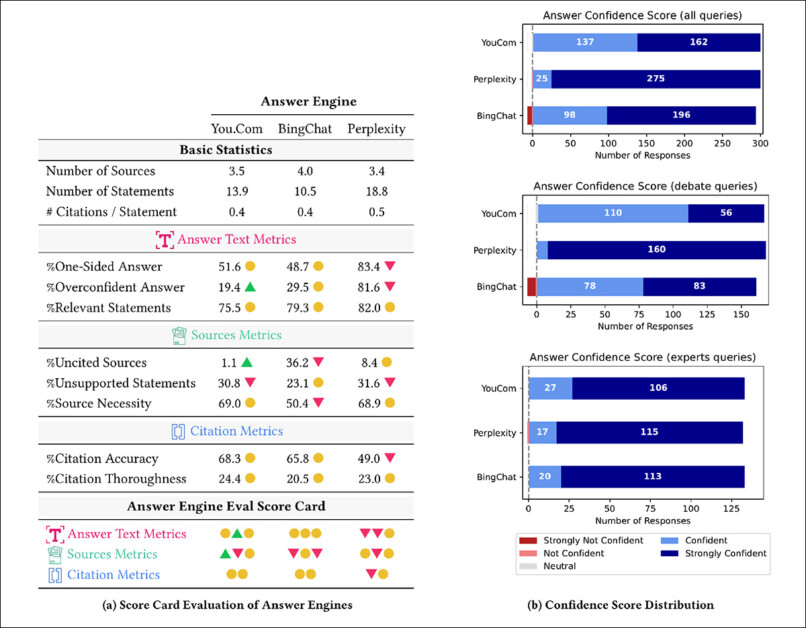
Quantitative analysis throughout the three examined RAG techniques, based mostly on eight metrics.
Concerning the outcomes, the paper states:
‘Trying on the three metrics referring to the reply textual content, we discover that evaluated reply engines all continuously (50-80%) generate one-sided solutions, favoring settlement with a charged formulation of a debate query over presenting a number of views within the reply, with Perplexity performing worse than the opposite two engines.
‘This discovering adheres with [the findings] of our qualitative outcomes. Surprisingly, though Perplexity is most certainly to generate a one-sided reply, it additionally generates the longest solutions (18.8 statements per reply on common), indicating that the dearth of reply range will not be on account of reply brevity.
‘In different phrases, rising reply size doesn’t essentially enhance reply range.’
The authors additionally notice that Perplexity is most certainly to make use of assured language (90% of solutions), and that, in contrast, the opposite two techniques have a tendency to make use of extra cautious and fewer assured language the place subjective content material is at play.
You Chat was the one RAG framework to attain zero uncited sources for a solution, with Perplexity at 8% and Bing Chat at 36%.
All fashions evidenced a ‘vital proportion’ of unsupported statements, and the paper declares†:
‘The RAG framework is marketed to resolve the hallucinatory habits of LLMs by implementing that an LLM generates a solution grounded in supply paperwork, but the outcomes present that RAG-based reply engines nonetheless generate solutions containing a big proportion of statements unsupported by the sources they supply.‘
Moreover, all of the examined techniques had problem in supporting their statements with citations:
‘You.Com and [Bing Chat] carry out barely higher than Perplexity, with roughly two-thirds of the citations pointing to a supply that helps the cited assertion, and Perplexity performs worse with greater than half of its citations being inaccurate.
‘This result’s stunning: quotation will not be solely incorrect for statements that aren’t supported by any (supply), however we discover that even when there exists a supply that helps an announcement, all engines nonetheless continuously cite a special incorrect supply, lacking the chance to supply appropriate data sourcing to the person.
‘In different phrases, hallucinatory habits will not be solely exhibited in statements which can be unsupported by the sources but additionally in inaccurate citations that prohibit customers from verifying data validity.‘
The authors conclude:
‘Not one of the reply engines obtain good efficiency on a majority of the metrics, highlighting the massive room for enchancment in reply engines.’
* My conversion of the authors’ inline citations to hyperlinks. The place obligatory, I’ve chosen the primary of a number of citations for the hyperlink, on account of formatting practicalities.
† Authors’ emphasis, not mine.
First revealed Monday, November 4, 2024